MOR SI CFP | Artificial Intelligence in Organizational Behavior and Human Resource Management Research: Opportunities and Challenges

CALL FOR PROPOSALS
Management and Organization Review
Special Issue on ‘Artificial Intelligence in Organizational Behavior and Human Resource Management Research: Opportunities and Challenges’
Guest Editors
Xiao-Yun Xie1, Long Zhang2, Lin Wang3, Kaifeng Jiang4, and Kai Chi Yam5
1Zhejiang University, 2Hunan University, 3Sun Yet-San University, 4The Ohio-State University, and 5National University of Singapore
Proposal Submission Deadline: September 25, 2024
Paper Submission Deadline: April 30, 2025
Special Issue Theme Background
Artificial Intelligence (AI), or algorithmic-based applications handling cognitive functions associated with humans, has become an indispensable part of modern organizations globally (Anthony et al., 2023; Benbya et al., 2020; Pietronudo et al., 2022). Diverse forms of AI, such as robotic process automation, deep learning algorithms, natural language processing, and computer vision techniques, not only empower managers with data-driven decision-making abilities (Shrestha et al., 2019), but also transform organizational capabilities and procedures (Borges et al., 2021; Raisch & Krakowski, 2021). Recently, generative AI like large-language models has attracted considerate attention (Grimes et al., 2023). This latest advancement challenges organization to align their strategies with the rapidly evolving field of AI,opening up new possibilities for problem solving, creativity, and work design within organizations (Anthony et al., 2023; Li et al., 2021; Paschen et al., 2020; Raisch & Fomina, 2023). For example, ChatGPT can generate new ideas and solutions in response to prompts provided by users (Budhwar et al., 2023). Therefore, the evolution and application of AI present fresh opportunities and challenges for strategic management, organizational behavior and human resource management (OBHRM), and other management and organization areas (Borges et al., 2021; Budhwar et al., 2023; Korzynski et al., 2023). This special issue, together with the special issue on ‘Opportunities and Challenges of Artificial Intelligence in Strategic Management and Organizational Innovation: New Theories, Perspectives, and Evidence’, seeks to delve into the myriad ways through which AI reshapes the landscape of management and organization research across various contexts, especially in China.
Existing studies about AI in OBHRM show that although AI has the potential to enrich work design (Parent-Rocheleau & Parker, 2022), enhance management capabilities (Anthony et al., 2023), improve employer-worker relations (Kellogg et al., 2020), and enable creativity (Jia et al., 2023), it also comes with challenges. For example, being exposed to AI can lead to increased employee job insecurity (Yam et al., 2023), reduced employee team identity (Savela et al., 2020) and well-being (Kinowska & Sienkiewicz, 2023). Whereas these insights are valuable, the literature remains largely silent on emerging issues stemming from the rapid advancement of AI capabilities. For instance, with the widespread usage of AI, the central role of ‘social beings’ in traditional organizational management has been weakened (Budhwar et al., 2023; Mariani, Machado, & Nambisan, 2023). Managers are faced with the challenge of not only effectively managing ‘AI employees’ but also facilitating interactions between humans and AIs (Curchod et al., 2020; Haefner et al., 2021; Nishant et al., 2020). Furthermore, unlike conventional technological tools, AI gradually undertakes both cognitive and physical tasks, thereby fundamentally altering the nature of work by changing both its content and execution methods(Raisch & Krakowski, 2021). Due to the invisibility, inscrutability and constant change of AI, tension arises about how human employees make sense of AI and develop skills to collaborate with AI-related technologies in their work (Anthony et al., 2023).
More intriguingly, beyond serving as a mere tool to assist human employees in their work, AI can also manifest itself in novel forms as team members (Jia et al., 2023; McNeese et al., 2021) or even assumes leadership roles (De Cremer, 2020). This presence of AI redefines team dynamics with respect to structural arrangements, communication patterns, and interpersonal relationships, and ultimately, team outcomes. Additionally, AI has progressively infiltrated various HRM procedures, increasingly assuming significant responsibilities such as layoff decision-making (Budhwar et al., 2023; Korzynski et al., 2023). Finally, current studies predominantly focus on examining the effects of AI at the individual level (Yam et al., 2023) and are largely conceptual (Malik et al., 2023; Raisch & Krakowski, 2021) or based on laboratory experiments (Ahn et al., 2022; Gonzalez et al., 2022). Such studies may not fully reflect the real-world complexities and practical challenges associated with implementing AI in real work environments and demonstrating the utility of AI-related technologies at the organizational level. This is particularly relevant in the context of China, where the use of AI has increased exponentially relative to other developed economies. Robot density, for example, has more than quadrupled from 2015 to 2020 (IFR, 2021). Likewise, extant research on AI has predominantly taken a WEIRD perspective (Liu et al., 2023), whereas cross-cultural perspectives of the application and perception of AI are largely missing (Yam et al., 2023).
Recognizing AI’s potential to reshape and refine the management discipline, the Business Administration Division of the Department of Management Sciences in the National Natural Science Foundation of China (NSFC) has consistently advocated for the integration of AI into management and organization research. Recently, a series of key projects of NSFC that focus on fundamental research and frontier topics in AI-driven management have been announced, aiming to propel the evolution of the management discipline. In alignment with these initiatives, this special issue aims to ignite a robust dialogue on how AI is transforming the research and practice of OBHRM, and what this means for the future of organization management.
The focal research question for this special issue is: What opportunities and challenges does AI bring to OBHRM theories and practices? Our aim is to explore how AI is transforming the organizational landscape, redefining work tasks and HRM practices, and potentially refining or challenging existing theories in OBHRM. More concretely, at the individual level, the primary goal is to understand AI’s impact on employee cognition, attitudes, and behaviors. At the team level, the main purpose is to examine how AI affects team processes, communication, coordination, and team outcomes. At the organizational level, the focus is on investigating the effects of AI on HRM strategy and practices, including but not limited to staffing, training, performance management, compensation, etc.
Scope of the Special Issue
This special issue invites research that enriches and/or challenges the current theoretical framework, empirical evidence, or practical applications concerning the impact of AI on issues related to OBHRM. We welcome a variety of scholarly contributions, including theoretical papers, conceptual reviews, and empirical papers adopting quantitative and/or qualitative approaches. Potential topics of interests include, but are not limited to:
- Individual Cognition, Attitudes, and Behaviors:
- Teams, Multiteam Systems, Communication, Coordination, and Leadership:
- Human Resource Management:
- Proposal Submission Requirements
1. We invite researchers to submit preliminary proposals for the special issue by the designated deadline (Deadline: September 25, 2024). Proposals should not exceed ten double-spaced pages (excluding references, tables, figures, and appendix) and should include the research motivation, specific research questions, a brief and relevant literature review, hypotheses, research design, and a proposed analytical plan. We welcome the inclusion of pilot data, although they are not required in proposal submissions.
2. Proposals should also include concise academic vitae of all authors, limited to a maximum of five pages each. The vitae should highlight the educational background, professional experience, and academic achievements of the authors.
3. Proposals should include a timeline for completing the project from the date of approval.
Special Issue Timeline
1. Proposal Submission (Deadline: September 25, 2024). Please send your preliminary proposals to specialissue@zju.edu.cn before the deadline, with the subject line ‘Proposal for Artificial Intelligence in OBHRM Research’. Please include all relevant materials in a single document.
2. Paper Development Workshop for Accepted Proposals (October 2024, School of Management, Zhejiang University). The authors of accepted proposals will be invited to participate in the workshop to further develop their research. Promising proposals will be encouraged to submit a revised version and to submit a full manuscript for consideration for publication in this MOR special issue. Please note that participation in the workshop does not guarantee final acceptance, it will be contingent upon subsequent revisions.
3. Paper Submission Deadline (Deadline: April 30, 2025). Papers for the special issue should be submitted electronically through MOR’s ScholarOne Manuscripts site at http://mc.manuscriptcentral.com/mor and identified as submission to the ‘Artificial Intelligence in Organizational Behavior and Human Resource Management Research: Opportunities and Challenges’. Participation in the paper development workshop is not a prerequisite for submission. We welcome all completed and relevant papers.
4. Publication of the Special Issue (TBD).
Special Issue Guest Editors
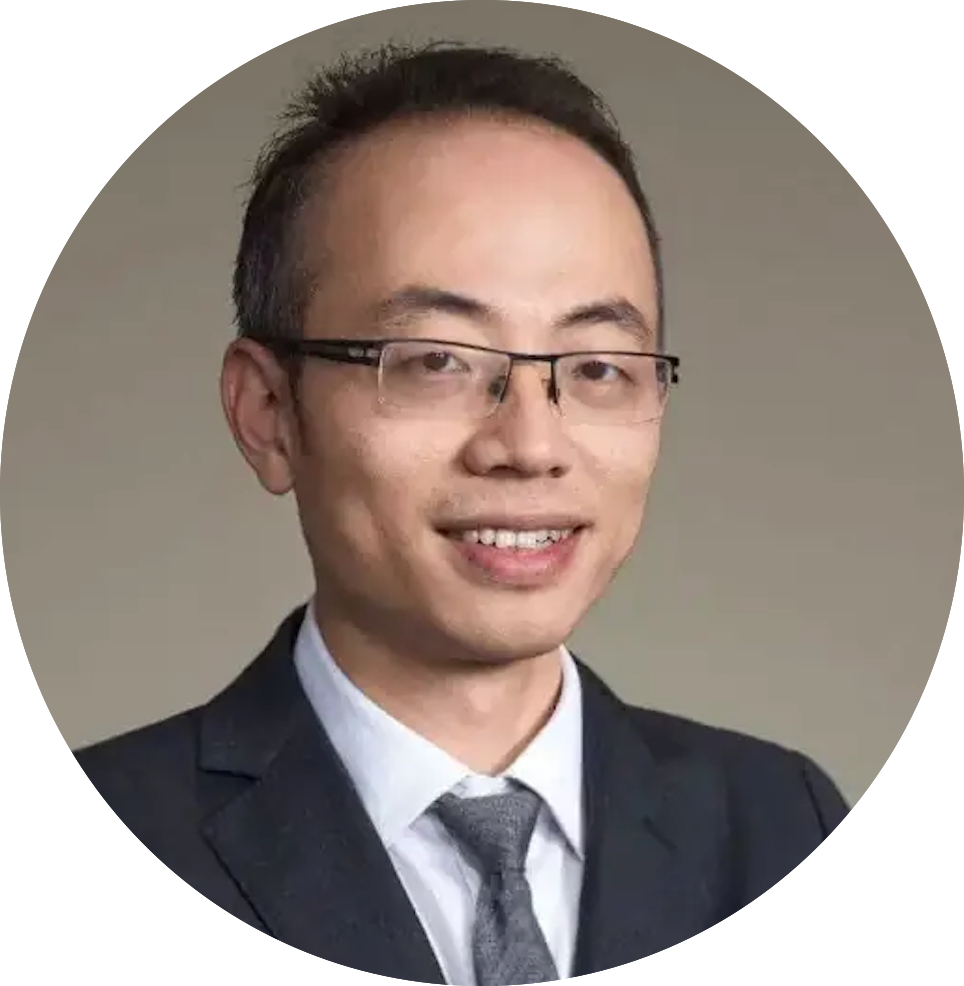
Xiao-Yun Xie (Email: xiexy@zju.edu.cn) is a full professor of Management at Zhejiang University. His current passion of research centers on organizational design, team boundary spanning, learning behavior, identification, and intra-group conflict under digitalization settings. He currently serves on the editorial board of multiple peer-review journals. He received his PhD from Zhejiang University in 2005.
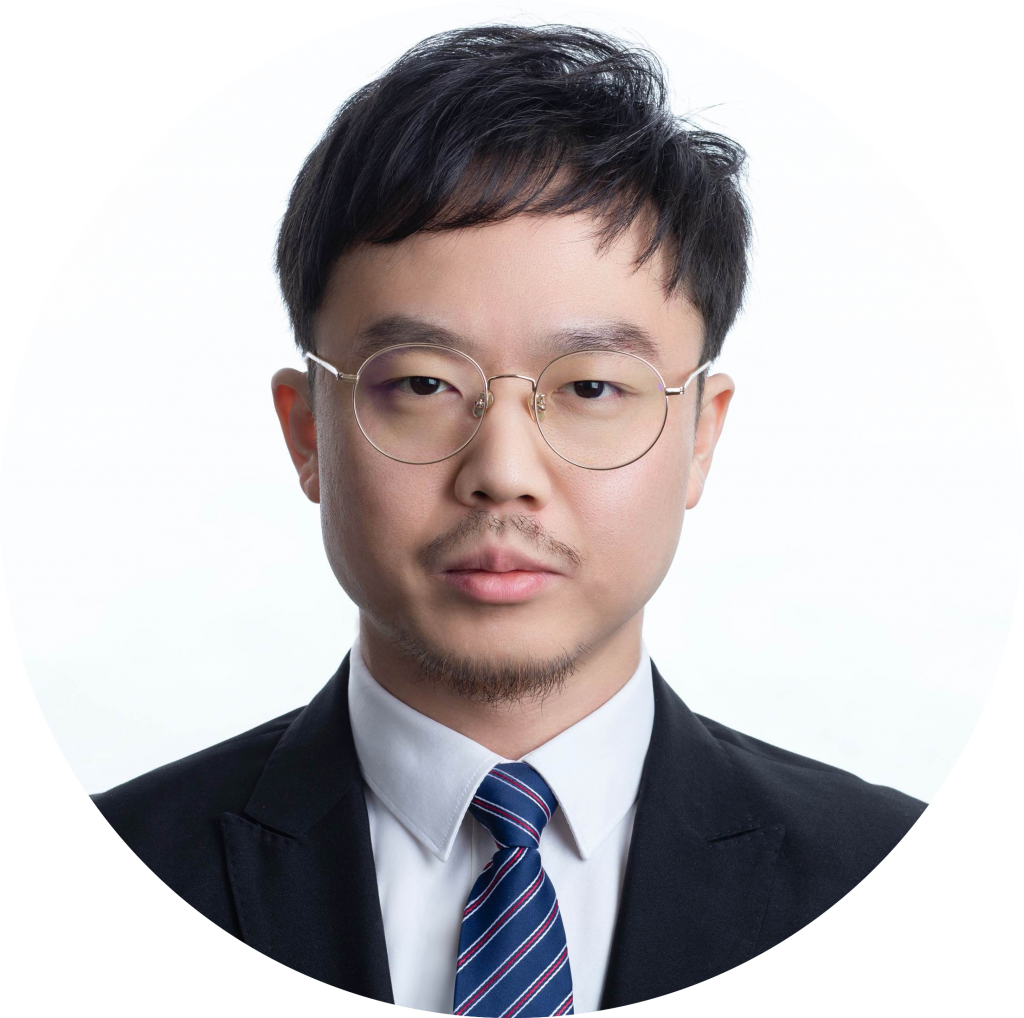
Long Zhang (Email: longzhang@hnu.edu.cn) is a professor in the Department of Business Administration at the Business School of Hunan University. His research interests include strategic human resource management, organizational behavior, innovation, and creativity. He currently serves as editorial board member and/or associate editor of multiple journals such as Management and Organization Review and Chinese Management Studies. He received his PhD from University of New South Wales in Sydney in 2017.
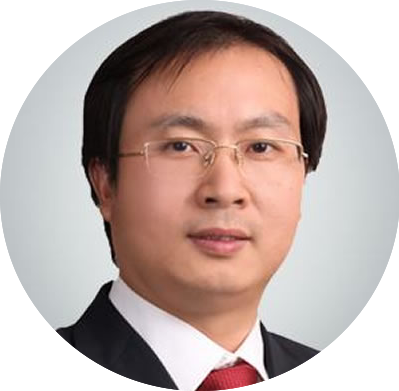
Lin Wang (Email: wangl83@mail.sysu.edu.cn) is a professor in the School of Business, Sun Yat-sen University. His research interests include leadership and team processes, proactivity, person-job fit and misfit, and newcomer adjustment. His research has been published in the Organization Science, Journal of Applied Psychology, Organizational Behavior and Human Decision Processes, Personnel Psychology, and Journal of Organizational Behavior. He currently serves as editorial board member of multiple peer-review journals. He received his PhD from Sun Yat-sen University in 2009.
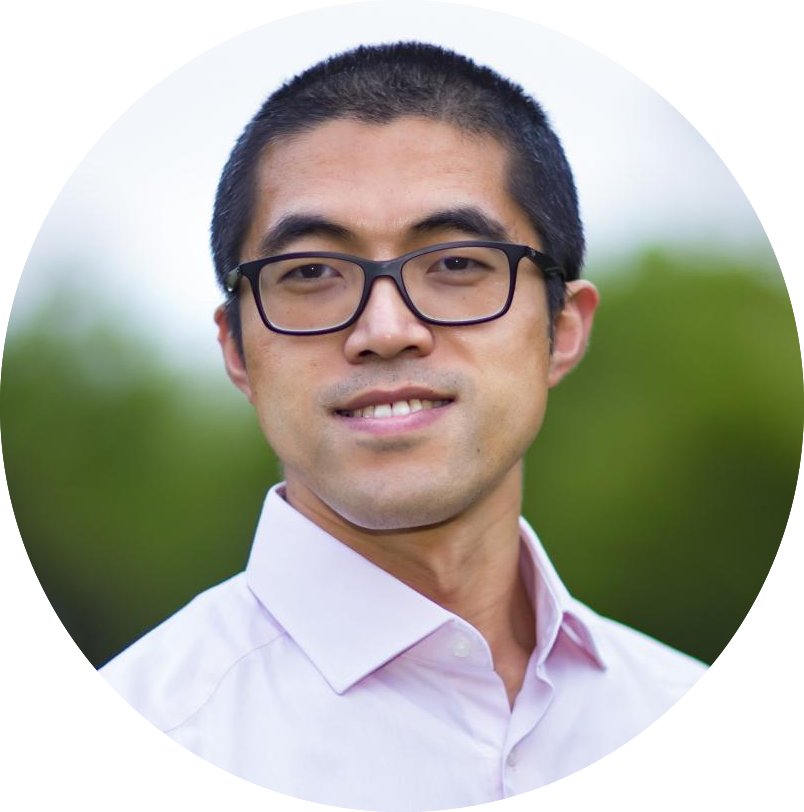
Kaifeng Jiang (Email: jiang.1788@osu.edu) is a Distinguished Professor of Management and Human Resources at The Ohio State University and a visiting Chair Professor at Guanghua School of Management, Peking University. His research primarily revolves around the impact of HRM practices and systems on employees, teams, and organizational outcomes. He ranks among the most productive and influential scholars in strategic HRM and has been rated as world’s top 2% of the most-cited scholars and scientists for three consecutive years (2020, 2021, and 2022) by Stanford University. He is a fellow of the Society for Industrial and Organizational Psychology, an Associate Editor of Personnel Psychology, and the Chair-Elect of the Human Resources Division of the Academy of Management.
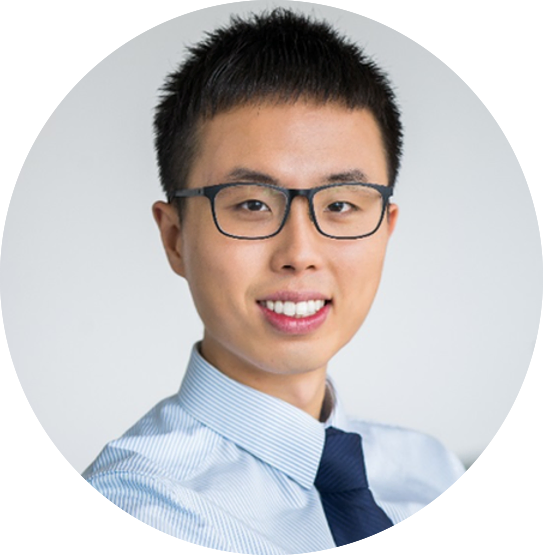
Kai Chi (Sam) Yam (bizykc@nus.edu.sg) is a Professor of Business Administration at the National University of Singapore. His current research interest is in human-technology interaction. He received his PhD from University of Washington.
REFERENCES
Ahn, J., Kim, J., & Sung, Y. (2022). The effect of gender stereotypes on artificial intelligence recommendations. Journal of Business Research, 141: 50–59.
Anthony, C., Bechky, B. A., & Fayard, A. L. (2023). ‘Collaborating’ with AI: Taking a system view to explore the future of work. Organization Science, 34(5): 1651–1996.
Benbya, H., Davenport, T. H., & Pachidi, S. (2020). Artificial intelligence in organizations: Current state and future opportunities. MIS Quarterly Executive, 19(4): 9–21.
Borges, A. F., Laurindo, F. J., Spínola, M. M., Gonçalves, R. F., & Mattos, C. A. (2021). The strategic use of artificial intelligence in the digital era: Systematic literature review and future research directions. International Journal of Information Management, 57: 102225.
Budhwar, P., Chowdhury, S., Wood, G., Aguinis, H., Bamber, G. J., Beltran, J. R., Boselie, P., Cooke, F. L., Decker, S., DeNisi, A., Kumar Dey, P., Guest, D., Knoblich, A. J., Paauwe, J., Papagiannidis, S., Patel, C., Pereira, V., Ren, S., Rogelberg, S., Saunders, M. N. K., Tung, R. T., & Varma, A. (2023). Human resource management in the age of generative artificial intelligence: Perspectives and research directions on ChatGPT. Human Resource Management Journal, 33(3): 606–659.
Curchod, C., Patriotta, G., Cohen, L., & Neysen, N. (2020). Working for an algorithm: Power asymmetries and agency in online work settings. Administrative Science Quarterly, 65(3): 644–676.
De Cremer, D. (2020). Leadership by algorithm: Who leads and who follows in the AI era? Harriman House.
Gonzalez, M. F., Liu, W., Shirase, L., Tomczak, D. L., Lobbe, C. E., Justenhoven, R., & Martin, N. R. (2022). Allying with AI? Reactions toward human-based, AI/ML-based, and augmented hiring processes. Computers in Human Behavior, 130: 107–179.
Grimes, M., Von Krogh, G., Feuerriegel, S., Rink, F., & Gruber, M. (2023). From scarcity to abundance: Scholars and scholarship in an age of generative artificial intelligence. Academy of Management Journal, 66(6): 1617–1624.
Haefner, N., Wincent, J., Parida, V., & Gassmann, O. (2021). Artificial intelligence and innovation management: A review, framework, and research agenda. Technological Forecasting and Social Change, 162: 120–392.
IFR International Federation of Robotics (IFR). (2021). Robot density nearly doubled globally. Available from URL: https://ifr.org/ifr-press-releases/news/robot-density-nearly-doubled-globally
Jia, N., Luo, X., Fang, Z., & Liao, C. (2023). When and how artificial intelligence augments employee creativity. Academy of Management Journal, 67(1). doi: https://doi.org/10.5465/amj.2022.0426
Kellogg, K. C., Valentine, M. A., & Christin, A. (2020). Algorithms at work: The new contested terrain of control. Academy of Management Annals, 14(1): 366–410.
Kinowska, H., & Sienkiewicz, Ł. J. (2023). Influence of algorithmic management practices on workplace well-being–evidence from European organisations. Information Technology & People, 36(8): 21–42.
Li, J., Li, M., Wang, X., & Jason, T. (2021). Strategic directions for AI: The role of CIOs and boards of directors. MIS Quarterly, 45(3): 1603–1643.
Liu, N. T. Y., Kirshner, S. N., & Lim, E. T. (2023). Is algorithm aversion WEIRD? A cross-country comparison of individual-differences and algorithm aversion. Journal of Retailing and Consumer Services, 72: 103259.
Malik, A., Budhwar, P., Mohan, H., & NR, S. (2023). Employee experience–the missing link for engaging employees: Insights from an MNE’s AI‐based HR ecosystem. Human Resource Management, 62(1): 97–115.
Mariani, M. M., Machado, I., & Nambisan, S. (2023). Types of innovation and artificial intelligence: A systematic quantitative literature review and research agenda. Journal of Business Research, 155: 113364.
McNeese, N. J., Schelble, B. G., Canonico, L. B., & Demir, M. (2021). Who/what is my teammate? Team composition considerations in human–ai teaming. IEEE Transactions on Human-Machine Systems, 51(4): 288–299.
Nishant, R., Kennedy, M., & Corbett, J. (2020). Artificial intelligence for sustainability: Challenges, opportunities, and a research agenda. International Journal of Information Management, 53: 102–104.
Parent-Rocheleau, X., & Parker, S. K. (2022). Algorithms as work designers: How algorithmic management influences the design of jobs. Human Resource Management Review, 32(3): 100838.
Paschen, U., Pitt, C., & Kietzmann, J. (2020). Artificial intelligence: Building blocks and an innovation typology. Business Horizons, 63(2): 147–155.
Pietronudo, M. C., Croidieu, G., & Schiavone, F. (2022). A solution looking for problems? A systematic literature review of the rationalizing influence of artificial intelligence on decision-making in innovation management. Technological Forecasting and Social Change, 182: 121828.
Raisch, S., & Fomina, K. (2023). Combining human and artificial intelligence: Hybrid problem-solving in organizations. Academy of Management Review, forthcoming.
Raisch, S., & Krakowski, S. (2021). Artificial intelligence and management: The automation–augmentation paradox. Academy of Management Review, 46(1): 192–210.
Savela, N., Kaakinen, M., Ellonen, N., & Oksanen, A. (2020). Sharing a work team with robots: The negative effect of robot co-workers on in-group identification with the work team. Computers in Human Behavior, 115: 106585.
Shrestha, Y. R., Ben-Menahem, S. M., & Von Krogh, G. (2019). Organizational decision-making structures in the age of artificial intelligence. California Management Review, 61(4): 66–83.
Wu, F., Lu, C., Zhu, M., Chen, H., Zhu, J., Yu, K., Li, L., Li, M., Chen, Q., Li, X., Cao, X., Wang, Z., Zha, Z., Zhuang, Y., & Pan, Y. (2020). Towards a new generation of artificial intelligence in China. Nature Machine Intelligence, 2(6): 312–316.
Yam, K. C., Tan, T., Jackson, J. C., Shariff, A., & Gray, K. (2023). Cultural differences in people’s reactions and applications of robots, algorithms, and artificial intelligence. Management and Organization Review, 19(5): 859–875.
Yam, K. C., Tang, P. M., Jackson, J. C., Su, R., & Gray, K. (2023). The rise of robots increases job insecurity and maladaptive workplace behaviors: Multimethod evidence. Journal of Applied Psychology, 108(5): 850–870.